Accelerating AI adoption: How insurers can stay ahead
Balancing customer expectations with regulatory requirements
For insurance organisations, customer experience matters more than ever. Customers have come to expect personalised touchpoints, omnichannel experiences and seamless end-to-end journeys, shaped by their interactions with digital native suppliers. Too often, their experiences fall short of those expectations, which has been highlighted by the FCA. This is particularly important for neurodivergent customers who may need suitable adjustments made so they can access their insurers' products and services fully. The FCA’s Consumer Duty has set higher and clearer standards for firms regarding customer needs:
"Firms must deliver good outcomes to customers while continually monitoring outcomes and evaluating them to ensure compliance. It is the responsibility of firms to define what good outcomes mean to their organisation and measure their progress with data."
While most insurers have implemented various FCA recommendations regarding Consumer Duty compliance, it is important to remember that customer experience initiatives take months or years to realise their full potential, so the time to invest is now. Insurers often discover that investing in customer experience drives operations efficiency and that these 'stacked wins' combine to improve the bottom line.
Artificial intelligence can help monitor customer journeys and outcomes by:
- Assessing large data sets and developing regulatory responses for internal and FCA reviews.
- Being proactive rather than reacting to regulations and market circumstances as and when they occur. This can drive new developments and create reflexive regulatory packages in insurance to aid compliance and customer facing teams in challenges in near real time.
- Showing how products are being managed and flag any regulatory issues
- Allowing for more wide-ranging reviews of policies, products and procedures as LLM models can be tested against policy documentation, regulatory guidance, and internal procedures to search for potential improvements.
- Assess the likelihood of a customer switching to another Insurance provider by analysing price rises in policies or services and supply mitigating information or a better assessment of the customer's needs.
- Identifying vulnerable customers within an insurer’s database and flagging this to ensure that their personal needs are met, whether this is through accessible documentation and technologies or interactive tools.
What are the other key use cases for AI in insurance?
AI can be adopted across any part of your insurance organisations, however the following core use cases can provide a higher level of impact on business performance:
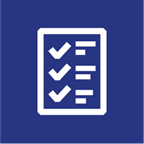
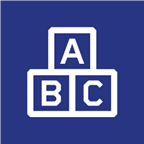
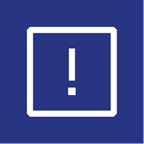
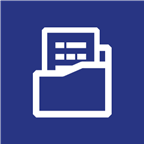
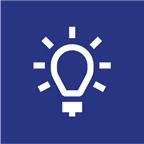
Accelerating AI adoption and keeping up with innovation
To convert AI aspirations into outcomes, Insurers must address these four major challenges:
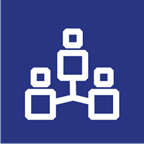
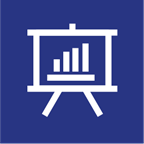
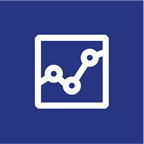
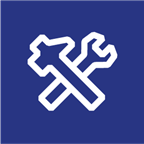
If you would like to understand more about accelerating AI adoption in your organisation and maximise its impact on your process, check out our Generative AI in FSI whitepaper: