Fight insurance fraud with data analytics
There are many technological pathways available when it comes to building your insurance organisation’s fraud strategy. It is however important to note that these technologies should not be deployed in isolation, but rather as part of an enterprise-wise approach to fighting fraud. Without an overhaul of company perspective about insurance fraud, honest customers could be turned away or deterred due to your strategy, with your newly invested technology becoming obsolete.
Data analytics is a potential route when building your insurance organisation’s strategy, through the analysis of patterns, anomalies and suspicious activities using data. The following methods are commonly deployed by insurance companies as part of their overall insurance strategy:
Anomaly detection
By analysing historical data and comparing it with real-time information, insurance companies can identify anomalies or deviations from the norm that may indicate fraudulent activities, including abnormal claim patterns, unusual behaviour by policyholders or agents, or atypical loss events.
Predictive modelling
Insurance companies can use predictive modelling techniques, such as machine learning algorithms, to identify patterns and predict the likelihood of fraud. By analysing historical data on fraudulent cases, these models can learn to recognise similar patterns in new data, allowing for proactive fraud detection.
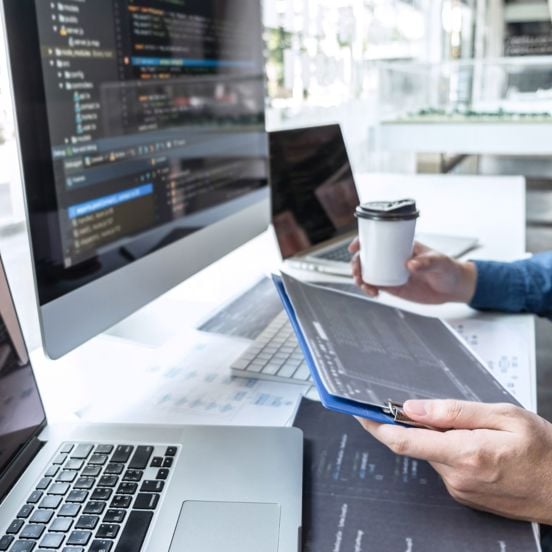
Social network analysis
Social network analysis involves mapping relationships between individuals, organisations, and entities to identify potential fraud networks. By analysing connections and relationships, insurance companies can detect suspicious clusters, collusive behaviours, or networks of individuals involved in fraudulent activities.
Geospatial Analysis
Geospatial analysis involves analysing geographical data to identify patterns and correlations. By mapping claim locations, policyholder addresses, and other relevant geographic data, insurance companies can identify clusters of suspicious activities, such as staged accidents or fraudulent claims in specific areas.
Link/network analysis
Link analysis examines relationships and connections between entities involved in insurance transactions. By visualising these relationships and analysing transactional data, insurance companies can identify complex fraud networks, identify previously unknown links, and detect suspicious behaviour.
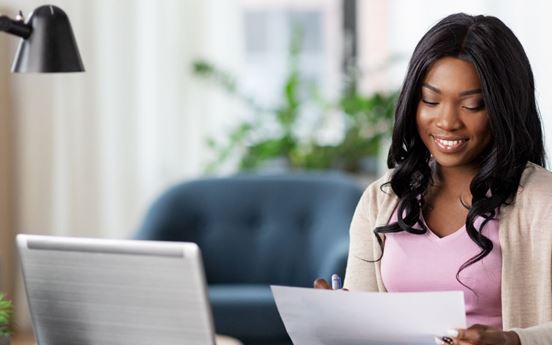
Text Mining and Natural Language Processing (NLP)
Text mining and NLP techniques can analyse unstructured data sources, such as claim descriptions, customer communications, or online reviews. Insurance companies can identify potential indicators of fraud or inconsistencies in narratives by extracting relevant information and applying sentiment analysis.
Image analysis
Making comparisons between the images submitted in supporting claims can reveal fraud indicators when compared to known policy/claim details and existing images.
Real-time monitoring
Implementing real-time monitoring systems allows insurance companies to analyse incoming data quickly and detect potential fraud in the early stages. Real-time monitoring can trigger alerts for unusual activities, high-risk transactions, or suspicious patterns, enabling swift intervention.
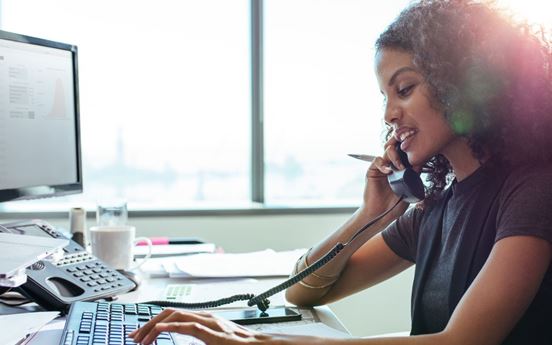
Collaboration and data monitoring
Insurance companies can share data with industry consortiums, fraud detection agencies, and law enforcement agencies. By pooling resources and data, insurers can leverage collective intelligence to identify emerging fraud trends and share information about known fraudsters.
While these methods can be highly effective in preventing insurance fraud, they do rely heavily on a high volume of quality data being accessible and the complexity of the analytical models and algorithms in use.
If you're unsure about the quality of your organisation's data, watch our recent webinar to learn from our experts on how you can get your data right.