Navigating the present to safeguard the future: balancing AI safety and security
On 1-2 November, the UK government hosts the world’s first global artificial intelligence (AI) Safety Summit at Bletchley Park. The summit has attracted publicity and conflicting responses on the scope. In this blog, we hear from David Haber, Founder and CEO of Lakera, John Sotiropoulos, Sr Security Architect at Kainos and Suzanne Brink, PhD, Data Ethics Manager at Kainos, as they discuss the summit and how it relates to the challenges we see in AI adoption. We propose a six-point action plan to help policymakers and organisations safeguard AI whilst grappling with the more complex questions.
The summit is held at a time when discussions around the EU AI Act are in full swing, and the White House signs its Executive Order on Safe, Secure, and Trustworthy AI. These discussions follow other international initiatives, such as the OECD AI Principles, the Global Partnership on AI (GPAI), the UN’s Advisory Board on AI, and the inclusion of AI safety in the 2023 G7 Summit in Hiroshima. None of these are surprising, given the dire warnings of pre-eminent AI scientists and tech industry leaders to pause and safeguard AI. AI safety seems to be an urgent concern both for policymakers and societies.
AI safety is one of the few AI trustworthiness-related terms not defined by the ISO/IEC 22989 standard on artificial intelligence concepts and terminology. The UK’s Department for Science, Innovation & Technology (DSIT) describes it as “the prevention and mitigation of harms from AI. These harms could be deliberate or accidental; caused to individuals, groups, organisations, nations or globally; and of many types, including but not limited to physical, psychological, or economic harms.”
Defined like this, AI safety can encompass a wide variety of risks. The recent discussion paper "Capabilities and Risks from Frontier AI", published by DSIT in late October, provides a detailed review of risks. Including;
- cross-cutting risk factors - lack of standards, concentration of AI market power, challenges in tracking AI and its misuse
- societal harms - degradation of the information environment, the disruption of labour markets, bias, unfairness and representational harms
- misuse risks - cyber attacks, disinformation campaigns, and dual-use science risks in fields like biotechnology and chemistry
- loss of control by humans over AI.
At the summit, the focus will be on misuse risks and loss of control risks related to narrow AI with dangerous capabilities (e.g., AI models for bioengineering) and frontier AI. The latter consists of highly capable models that can perform various tasks and match or exceed the capabilities of today’s most advanced models. As part of this focus, cyber security is expected to be central to the discussion. Both because proper security of AI models can help prevent adversarial misalignment due to attackers and because cybercrime is a form of misuse that can be made easier by advanced AI.
The rapid adoption of AI makes prioritising misuse and security a sensible choice. With almost all major organisations looking to deploy generative AI to production within the next months, these discussions are urgently needed.
Time is of the essence, however, and policy-making cycles take time. Alongside these important discussions, we therefore need to urgently put principles into practice today. This includes some immediate practical measures:
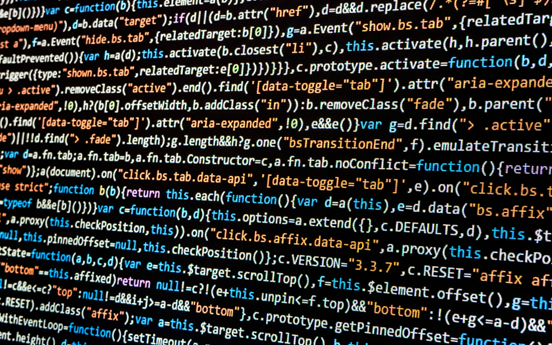
1. Increase testing and assurance
Foundation model providers must adopt proactive measures such as red teaming and comprehensive testing before releasing their models to the public. Recent discussions at the EU Parliament showed that high regulatory uncertainty exists, particularly around open-source models, so in the meantime, these requirements must apply to both proprietary and open-source models. Transparency on data and methods, as well as diversity of teams, will help mitigate the risks.
Organisations positioned to fine-tune and deploy pre-trained foundation models must also recognise their role in the chain of defence to red team and monitor advanced AI models as they continue to become available. Companies of all sizes must be put in a position to test and audit AI at an application level, with minimal overhead. Similarly, we need to apply a dual-lens approach to testing and apply it from the application side whilst equipping users to understand their residual risk.
2. Adopt actionable open standards
We must enable application builders to secure AI at the application level. Generative AI has blurred AI and application development with API-driven integrations, adding new risks to traditional AppSec challenges. It has also created an entirely new set of AI-specific risks, such as prompt injections and poisoning, and it has heightened the risk of pitfalls like overreliance on AI. Whilst policymakers are now beginning to talk about security standards, Open Standards organisations such as OWASP are at the frontline, developing concrete standards to help address both old and new risks. The OWASP's Top 10 for LLM Applications is an example of an actionable standard available today to protect from adversarial misalignment. With the focus on actionable verification, these standards complement official standards such as the ones published by ISO and BSI.
3. Accelerate standards alignment
The realisation of the urgent task to safeguard AI and the lack of mature standards have led to many standards initiatives. This is a positive development and helps highlight the task's urgency. However, there is a risk of conflicting taxonomies, contradictory vulnerability reporting, and inconsistent defences, which could confuse and undermine those at the frontline of AI development. Discussions are already taking place between OWASP, NIST, MITRE, CSA, and others to align and respond together to a rapidly evolving landscape of threats. In the UK, initiatives such as the AI Standards Hub help navigate the standards landscape. Further engagement with open standards organisations and the active fostering of alignment activities are key enablers, as we see various security challenges emerge like nightshade and its use of poisoning, the emergence of privacy inference attacks on LLMs, and questions around the theoretical limits of guardrails. These can only be responded to effectively with close collaboration.
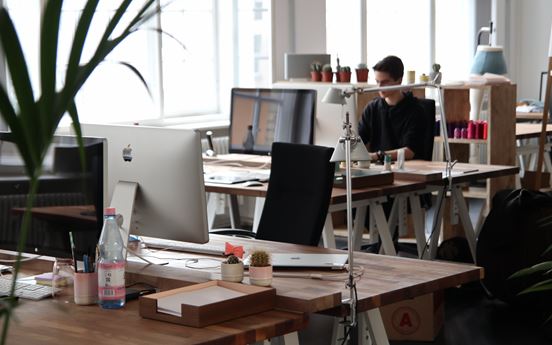
4. Invest in automated defences
Standards and mitigations have little value if they are hard to implement and enforce. A new breed of AI security vendors has emerged, specifically targeting LLMs and frontier AI. Companies like Lakera offer tooling that aligns with the OWASP Top 10 for LLM and provides API-driven automation to rapidly test and secure LLM applications – covering major risks from prompt injections to hallucinations and toxic language outputs. There are advantages to aligning with actionable standards. Vendors benefit from the research and updates feeding into the standards and their users benefit from well-defined concepts and taxonomies, verifiable controls and compliance reporting.
5. Integrate security with ethics
AI security can no longer remain in its traditionally neutral realm of protecting 'resources'. An analysis of 106 AI incidents between 2011-2022 found that 50% of cases were related to privacy incidents, 31% to bias, 14% to explainability with the remaining 5% being difficult to classify. While admittedly, these statistics might look different in future years, with the landscape rapidly shifting from narrow to more general AI, we cannot ignore the immediate safety risks of the AI models we deploy today. This includes the risks that AI-enabled services lead to (more) inequality and AI recommendations are accepted in critical contexts without understanding the model's logic.
A system that is produced securely but generates hateful and discriminatory outcomes is not a system safe from harm. We see this realisation being increasingly embraced by the AI security community, with the introduction of Overreliance and Excessive Agency in the Top 10 for LLMs and the appearance of safety checks (e.g., bias or hateful content) in the new generation of AI Security Tooling. We strongly believe that security needs to be combined with a data ethics framework in developing and deploying AI; this is essential to ensure that the full range of AI risks is managed, including risks around bias, transparency, and accountability.
6. Promote secure-by-design and ethics-by-design AI delivery
None of the previous points matter if they are not integrated into project delivery. Delivery must intentionally treat AI security and safety, starting with threat models and risk assessments. Companies like Kainos delivering AI using ethics-driven frameworks integrated with AI security and secure-by-design methodologies provide an example of how to utilise standards, ethics, and AI security tooling to address the AI safety challenges in action and not as an afterthought.
The six measures outlined in this blog constitute an immediate response to safeguard AI but are not the only course of action. They are essential safeguards. Debate and interventions to address the broader risks need to continue to take place alongside this, to make sure that we can address the long-term challenges, safeguard the immediate, and ensure ethics drives what is acceptable beyond traditional notions of what is secure.
Our People