Q&A with Kainos’ new Data Ethicist - Dr Suzanne Brink
Can you give us a bit of background on yourself and how you got into the field of Data and AI ethics?
The role of ethics in society has always been something I’ve wanted to explore. At university, I minored in philosophy and my PhD in psychology at Cambridge explored moral emotions and social comparison. Specifically, I looked at how people respond to others out-performing them in moral domains – such as an extreme act of bravery. What I found was encouraging. People are inspired by others who perform well in the moral domain. They want to emulate them. This shows how important it is to share stories of people who set the right example.
I have pursued this interest in bringing a positive impact to society into the workplace – spearheading Diversity, Equity, and Inclusion projects for a global corporation, and working as a psychology consultant at a leading recruitment technology company. It was in this latest role that I really started to think about the crossover between data, technology, and ethics.
I believe in the power of data. My background in psychology has taught me that humans often function by making snap judgements – we do not have time for contemplation every time we make a decision. We do a lot on autopilot and our intuitions can be misleading. For example, someone you are interviewing for a job may reveal they have a similar hobby to you. This fact is irrelevant to how they will perform in the job, but the rapport may bias you towards that person. I believe that data can help us challenge ourselves and apply a critical lens of objectivity to our decision making.
For me, this role is a great meeting of my enduring interest in ethics and my belief in using data for good decision making.
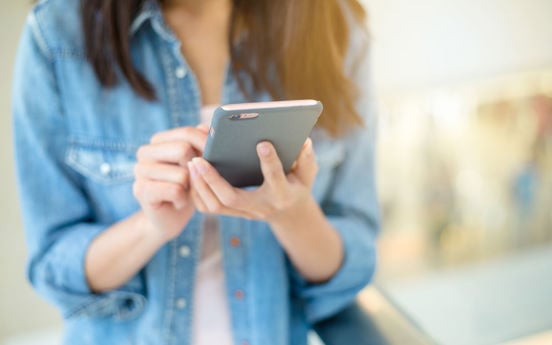
What will your main area of focus be at Kainos?
Responsibility and ethics are already a big part of the Kainos strategy, and the company has the right kind of energy and motivation around this space. Through ongoing data and AI projects, we have already built a strong foundation for tackling issues around bias, data quality and ethical design. My role will be to expand on what we already do, sharpen our offering, and structurally embed ethics in the delivery of our data and AI projects.
The role of the data ethicist is a relatively new field. What is driving interest in this space and what does ethical / responsible AI mean to you?
We must recognise that when we are modelling society, we are often modelling a world that is unfortunately not free from bias. AI is a technology that relies on data, and this data can carry these biases inherent in our society. So, questions need to be asked to ensure solutions promote fairness and equality.
Responsible AI can mean building tech “for good”. Imagine, for example, an algorithm that can predict areas most at risk from the impact of climate change and promote early intervention. However, many AI solutions are more neutral in intent. In those cases, responsible AI or data ethics rather refers to asking questions about how we build and deploy the technology in a way that has the most positive impact – or at least does the least harm. Questions include: How does the algorithm impact people’s thinking? Who is accountable if something goes wrong? Are there checks and balances to account for bias?
There are many different AI ethics frameworks available today. Research by Anna Jobin and colleagues has shown that typically, such frameworks include five key themes: fairness and justice, responsibility, privacy, transparency, and non-maleficence. Robustness is also important, and sustainability is becoming a more frequently heard dimension as well. All these components matter when it comes to data ethics, in my opinion.
In the end, responsible AI for me means both considering risks when developing AI and leveraging the opportunity to have a positive impact with AI – and there are many opportunities! The role of data ethicist is to challenge assumptions, ask questions and bring tools to support responsible development and implementation of the technology. Kainos is certainly forward-leaning in creating this role.
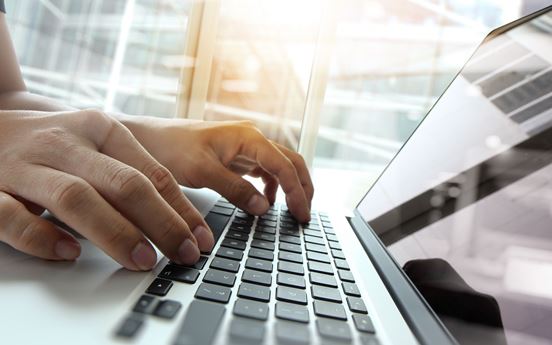
How can we ensure that inclusivity and fairness is built into AI models?
Inclusivity is key when developing technology. Ideally, this starts with the diversity of the team. If the team are all from the same milieu and background, they are likely to have the same blind spots. Equally, when building an AI model, the datasets used must be as representative and pluralistic as possible to set the right basis for a fair model. However, even with a balanced dataset, it may still be necessary to mitigate bias when building the model and one should consider monitoring for bias once the model is live as well.
Fairness is a particular issue in industries like recruitment where humans have traditionally brought bias to the process. Studies have shown that candidates can be judged solely on perceptions around their names. The moment you start building algorithms on such data, you need to be aware of bias and consciously tackle it.
Technology can do a lot, but it’s not magic. AI can amplify bias if the right checks are not in place, so humans must remain in the loop at the right stage. Getting this right takes investment and time.
What about big ethical questions in the industry?
Many of the questions we ask when designing data and AI solutions are actually very fundamental questions. How do we define success? How do we define fairness in this situation? Who do we hold responsible for the outcome if something goes wrong, and how can we properly explain what we have done and why we are doing it? And how do we avoid harmful, unintended uses of our processes?
I love that while we are rightfully excited about the innovation AI can bring, we also take the time to think about these core matters. And it is so important that we do, as we are hardwiring the society of tomorrow.