Trust and AI in the public sector: A problem of data engineering, not just data science
It has been long understood that Artificial Intelligence (AI) has the power to transform the public sector and public bodies are increasingly incorporating AI into their digital toolkit for the betterment of citizen services.
AI has been shown to reduce costs and improve efficiency of public sector services. For example through the automation of repetitive tasks, such as document comparison and review. It is also helping to increase the effectiveness of public sector services to deliver better outcomes, for example, through predictive capabilities to spot potential fraud before it occurs.
This is just the tip of the iceberg. There are countless ways in which AI could help, to not only improve, but transform public sector internal operations and citizen-facing services; going beyond efficiency gains to support better decision-making around everything from infrastructure planning to restoration and justice.
Yet for the public sector to embrace the unrealised potential of AI, it must be harnessed in an ethical way that protects citizens from potential misuse or bias. Realising this ambition is not simply a technology problem – arguably, the technology is already there to deliver these benefits.
The biggest roadblocks are two-fold:
- Firstly, data accessibility and quality; ensuring that data is stored in a centralised and accessible location and is cleansed, so that it is usable, reliable and trustworthy.
- Secondly, creating the right frameworks and sets of governance to safeguard that data is being used in an explainable, transparent, and ethical way.
In this two-part blog series, we will discuss these issues, setting out advice on how public sector organisations can forge a path ahead with AI.
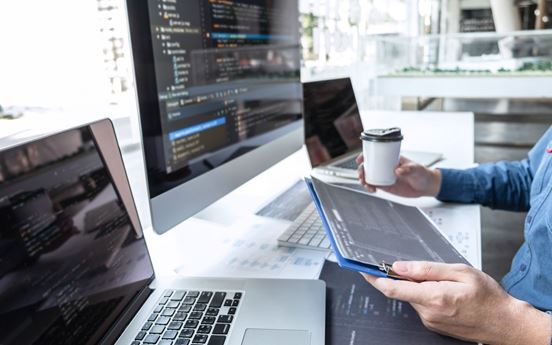
A data engineering problem, not just data science
When discussing the AI skills gap, the conversation largely focuses on the need to build knowledge and skills in the roles of data science and artificial intelligence. Yet while this is critically important to continued digital innovation and our future economic growth, such skills alone will not enable public sector to move forward with their AI ambitions.
Our experience of leading large-scale data science programmes of work into the public sector has shown that the need for these skills is negated by the even greater need for data engineering expertise – i.e. the upfront gathering, cleaning, collating, and harmonising of public sector data. This critical foundational process ensures data readiness for the application of AI and machine learning models and the importance of it cannot be underestimated.
The public sector plays a pivotal role and is a leading voice in making AI work for innovation, growth, and public value. However, it also faces huge hurdles to building effective services that leverage AI:
- The sheer scale of datasets can lead to fragmentation, silos and inconsistency between departments, local authorities and even teams. That makes it that much harder to surface the data needed, in the right format, to make the right decisions.
- Data collection and capture in the public sector can also be problematic. There is little consistency, which makes analysis difficult. And although we are well over a decade into the “Digital by Default” agenda set out by the Government Digital Service, many public sector services and associated datasets are still not digitally available — think historic patient records or physical citizen documents stored in filing cabinets. It means that generating a single view of a citizen in a single department is still a very real hurdle.
- Data bias can also be endemic in certain parts of the public sector. And for this or other historical reasons, demographic data is often not aligned with operational data, meaning the data that is accessible, isn’t contextualised to the point of being usable without enrichment from other data sources.
Engineering smarter public services
Before the public sector can begin to realise the true benefits that data science and AI can offer with the vast quantity of data that it holds, it needs to clean, harmonise, and make that data interoperable at scale.
To achieve it, the public sector must ramp up its data engineering capacity and capability. This will require a combined effort of internal upskilling, recruiting more practitioners and working closely with data transformation partners like Kainos who bring end-to-end technical excellence in data engineering, data science and AI.