Uncovering the challenges of making AI work for the Defence sector
It’s difficult to name an industry where artificial intelligence (AI) technology wouldn’t add value if developed and deployed in the correct manner. The Defence sector is certainly a prime candidate for modernisation in this way – a fact the UK government recognised in its recent AI strategy paper.
But perhaps more than in any other part of government, they must proceed with extreme caution – especially when AI is being used to deliver military effect, rather than back-office processes.
To succeed in this space, the Defence sector must overcome many challenges to ensure the integrity of AI outputs. It will fundamentally require excellence in data hygiene, the right level of access to high quality data, ethical and governance considerations, and close collaboration between diverse skill sets.
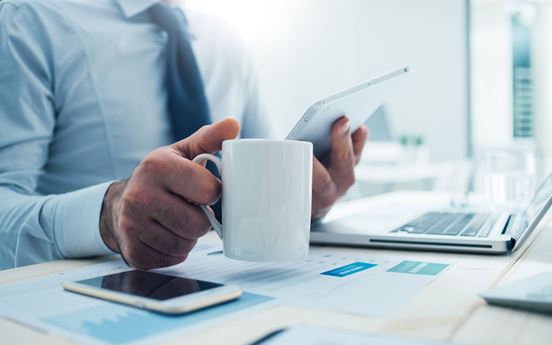
A huge and complex organisation
The Ministry of Defence (MoD) is a government department in name, but that’s where much of the similarity with the rest of government ends. There are around a dozen discrete parts – including the Army, Navy and Royal Air Force – with many enabling organizations, including new Kainos client, the Defence Science and Technology Laboratory (Dstl) and their part in the newly formed Defence AI Centre (DAIC).
Each of these organisations has its own budget, hierarchy, processes and policies, all of which operate under an overarching set of MoD policies.
Next, consider the scale of these departments. There are in the region of 194,000 serving members of the armed forces, 58,000 civil servants and countless contractors. The MoD also manages over 20,000 primary pieces of equipment – think aircraft carriers, fighter jets, main battle tanks (MBTs), and everything in between – and around a million individual stock keeping units. To put that in perspective, a large supermarket chain manages in the region of 90,000.
The bottom line: this is a vast, complex organisation operating across more than 40 countries, with multi-layered responsibilities and outputs – not to mention extra layers of administration for liaising with NATO and other Defence departments around the globe.
Unsurprisingly, this complexity brings with it a range of challenges when introducing new technology and – with AI – the ethical considerations of how it will positively and negatively impact people.
Unpicking the challenges of data and governance
These challenges are multi-layered and manifold.
The MoD has many highly skilled, incredibly intelligent and effective people. However, AI is a new frontier for everyone, maturing at a dizzying pace.
Take Dstl. Its mission is to develop cutting-edge capabilities for the Defence landscape of tomorrow. Alongside the exploration into new technologies and applications of AI in Defence, it is critical that the end-to-end model for delivering AI outcomes matures at the same pace, including innovation, exploration, people, teams, and organization. The MoD has set off on a fast-moving train and needs to ensure the track is laid ahead of it as they go – in terms of policy, assurance, and end-to-end management.
There are other cultural and organisational challenges to consider. Because the MoD traditionally focuses its procurement processes, governance, and approach around large-scale equipment or infrastructure programmes, it needs to re-gear for the fast turnaround times needed for AI projects.
Historical procurement processes also mean there can be a challenge getting hold of trustworthy data in the right volume to appropriately train AI models. We’re talking about siloed, federated systems of record across the MoD and supplier networks, which make it hard to generate a single source of truth and consistency. Data hygiene is a critical consideration.
Accessing that data can also be problematic for private sector partners, like Kainos, due to legitimate MoD security constraints and policies. Then there’s the classification system. Dataset A and Dataset B may separately both be unclassified, but when brought together could create a classified dataset only accessible to those with the right security clearance.
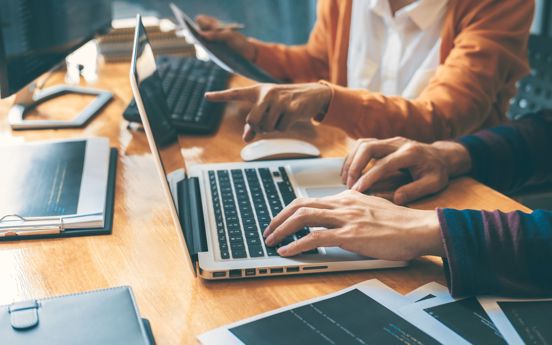
Delivering an AI-centric future
At Kainos, we’re tremendously excited to be working on some of these challenges with Dstl. We’re taking ideas, thoughts and solutions from some of the brightest minds in Defence technology and exploring these concepts through a business and ethical lens. In our next blog, we will examine some of the learnings we have taken from this work around how to implement AI in an ethical way within Defence and the safeguards that need to be applied.