Beyond Boundaries S1 E4 - Adopting AI: Building AI-ready cultures
Adopting AI: Building AI-ready cultures
In this episode of Beyond Boundaries, Gareth Workman, Kainos' Chief AI Officer, is joined by Rory Hanratty, Chief Technology Officer at Axial3D, to explore one of the most overlooked - yet essential - enablers of AI success: culture.
While technology may drive AI forward, it’s people who determine whether it truly takes root. From shifting mindsets and overcoming fear to aligning AI initiatives with business outcomes, Gareth and Rory discuss what it really takes to build AI-ready cultures that empower teams, inspire trust and scale innovation.
So how do leaders move beyond the hype and create the right environment for adoption? What role does curiosity play? And how can businesses ensure AI is solving real problems - not just chasing trends? Join us as we unpack the human side of transformation and offer practical guidance for organisations at any stage of their AI journey.
The full episode transcript is available here.
Watch here:
Listen here:
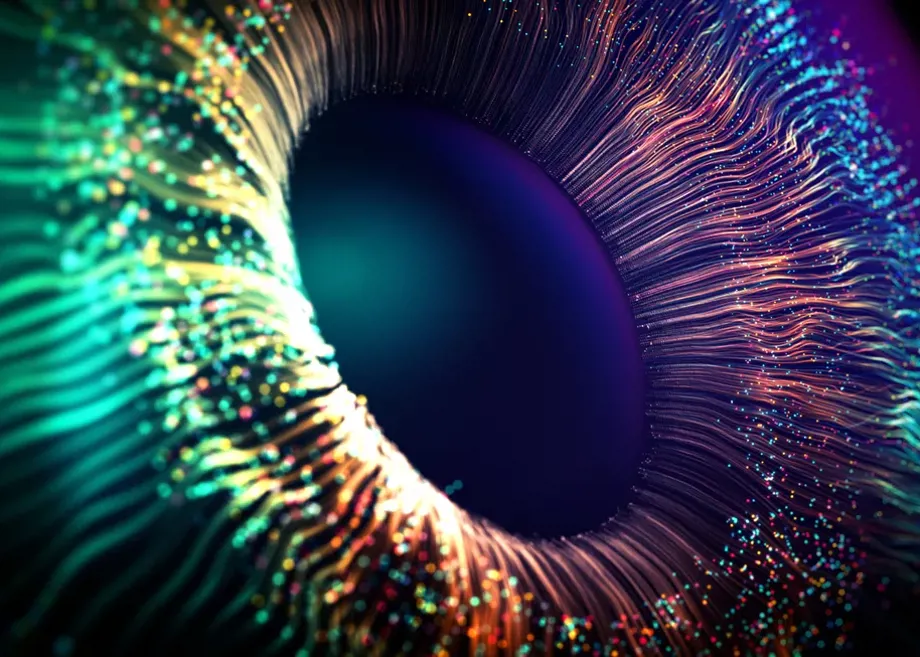
Sign up for episode reminders and exclusive thought leadership
Transcript
Teaser
Rory Hanratty
Give me a real example that I can point at. And then, when I'm talking to somebody else in another part of the organization, instead of going: 'we're gonna improve productivity by using large language models' and nobody knows what that means. You can say: 'we have reduced the time that it takes to write reports on customer satisfaction by this amount, or we're able to discover this new information about how our users use our system' or whatever it happens to be. When it's real and tangible and it's an outcome that matters, that's when you'll see people starting to go, right. This means something to me. This means something to us. We could do amazing things with this.
Start of episode
Gareth Workman
Welcome to Beyond Boundaries, the podcast from Kainos that helps business leaders navigate the fast evolving world of AI. So I'm Gareth Workman, Chief AI Officer at Kainos, and today we're exploring what's often overlooked, but absolutely critical to AI success, and that is your people.
Because here's the reality, no matter how advanced the technology, if your culture isn't ready, AI won't take root. In this episode, we'll unpack what it really takes to build AI ready cultures, ones that inspire trust, drive adoption, and empower teams to innovate with confidence. From shifting mindsets to overcoming fear, this conversation is about preparing people, not just systems for the future of work.
So joining me is someone who's seen firsthand how culture can accelerate AI success: Rory Hanratty, Chief Technology Officer at Axial3D. Rory, it's brilliant to have you with us. Welcome to Beyond Boundaries.
Rory Hanratty
Thank you Gareth, good to be here.
Gareth Workman
So I'm just going to dive straight in, Rory. So obviously, when you and I have spoken in the past, you've always highlighted the importance of being outcome-focused when it comes to AI. So what tangible benefits does it unlock when that mindset is applied?
Rory Hanratty
So yeah, I think, you know me from over the years, Gareth, is that my relentless focus is on users and outcomes and technology is always sort of a background to that. And I think if anyone's worked in engineering over the years, I'm sure they'll have had an experience with someone who is technically brilliant and is very much focused on the technology and not on an outcome.
And while you'll end up with something that is like a kind of almost like a cathedral to how great technology can be. It actually solves no problems. So if you don't have as a foundational part of your organisation the idea that we're focused on solving problems or delivering outcomes for people, you're just going to be building technology for technology sake. I guess a way to frame that, is that with, if you think about an AI system, anything that uses machine learning, we're getting towards this idea of agents doing work for us and all this other kind of stuff.
Imagine what sort of a world we're reflecting if no human is ever involved in what it is that you're doing. Like what's the value of what you're automating there? Like what is the point of what's happening? It becomes this kind of like system that's running on rails by itself. And if that's the case, actually, what have you built there? You've kind of done something that possibly has no value to anyone. Now, it's a bit of an extreme way of thinking or talking about it, but that's actually something to bear in mind in this, is that whether you're automating backend processes or you're building something that helps somebody with productivity, it's gonna touch a person somewhere in some way, shape or form. Start from there is usually my advice on this kind of stuff.
Gareth Workman
Fabulous. As you say, this will have impact to humans somewhere along the line. And even if you have a picture up or something to motivate that mindset of why you're doing it.
Rory Hanratty
Yeah, yeah, yeah, yeah. One of the great ways of trying to frame this as well, I always think is trying to map out the world in terms of where does AI fit in? What are you trying to get it to do? One of the ones that I always quite like leaning towards is the idea of the Wardley maps, which is putting the user right up at the top of the map and then identifying what are all the different things that you need to do to achieve the outcome for that user. And it anchors you in the user's world, which, yeah, like I'm saying, is incredibly, incredibly important there.
That's not to say that there isn't activity and investigation that needs to happen inside an organisation that isn't directly solving the user problem. One of the things I think that's particularly relevant now with AI and because of how quickly it's, I guess, increasing in terms of capabilities or what you're being told it's increasing in terms of capabilities, is that you need to build in this kind of part of your culture that's curious and actually accepts the idea that you might not get to an answer that you want. It's very different to implement in software technology where if I have somebody work on some code for two weeks and we plan to release a feature, we're more than likely, we've got good chance of success of getting that as a feature. If you start trying to build a model or use an LLM or using generative AI to get to an outcome, it is not guaranteed you will achieve that outcome. And that's a slightly different mindset that people have to bring into their organisations as well, is that actually 'r', the 'r' part of research and development, so R&D, the 'r' part of it becomes a little bit more important in terms of you frame that and how you work on those things.
Gareth Workman
Understood. So, in terms of, if you look broader, what are those cultural qualities or behaviours you see in organisations that are genuinely ready to adopt and harness AI successfully?
Rory Hanratty
So I think it's probably worth putting a little bit of context around the use of AI inside an organisation, right? And that's actually really, really important. That's behaviour one you need to have. To be contextually aware of what you're trying to do, right? So adoption of AI for a marketing company is going to be very different to adoption of AI for a software development company, versus a manufacturing company, versus us as a medical device company. So being contextually aware is super, super important. You should be having those conversations at a very senior level, clearly understanding what we are in the business of. Back to outcomes again, what is that we're trying to achieve by any use or implementation of technology and how do we get to those outcomes? So contextual awareness is a big thing and it might seem strange to say that for people who have spent a little bit of time in many different industries like.
That's my life, right? I've bounced around all these different industries and I'm always, every single time I step into somewhere else, I have to learn about that new industry, what matters, what are the kind of influences, how we achieve outcomes. But if you spend your entire life working in, let's say, manufacturing, that's your world and you are amazing, amazing at manufacturing. AI's turning up with a completely different context and an awful lot of hype and an awful lot of like, kind of, nuances to what you need to do.
So, you need to be able to interpret what it's offering in your own context. So that's behaviour one, being contextually aware and how can you get the most out of the technology that you're looking at? I mentioned the other thing as well is this idea of curiousness and having a strong capability in and around research and being able to scope research projects to evaluate technology to make sure that it will provide the outcomes that you need.
This used to be, I suppose, the kind of world of the, like the CIO, in the early days of cloud - the person who's wanted to do a big migration of a workload to the cloud, usually CIO, because you're costing us money by having data centres. So, you need to create the business case. You need to figure out what impact it's going to have. How is that going to directly impact either bottom line or the number of people that we have and all that kind of stuff. And it was a kind of straightforward bounded problem that you needed to solve. And in that role, you'd learn the skills that you needed to learn around cloud and it would be your problem, and your CEO could kind of basically know nothing about cloud really. CIO, that's your problem, go off and fix it.
Well, AI is turning up with something very, very different, which is it impacts every part of your business in different ways. So being able to, as let's say, the CFO, understand and scope how I might use AI to increase efficiency in my financial operations. Our CIO, how do I use it to, kind of, improve my security posture or whatever it happens to be like that, kind of little story repeats over and over and over again.
So those skills of being able to evaluate technology, to scope up projects, to figure out how can this impact me directly becomes almost like a cross-cutting concern for everyone. That wasn't the case before when you're talking about maybe digital transformation or software or whatever. It was a little bit more niche, but yeah, that's fascinating about what's happened with this actually.
Gareth Workman
I really like your piece about bringing your domain expertise, almost your superpower to that context to the situation. So one of the things I'd be curious about from your experiences, where do you see companies most often stumble? Maybe it's not in the tech space, but whether it's mindset or other things, where do you think is the bit you see them really struggle to get to grips?
Rory Hanratty
Good one. So, I'm probably going to talk about this from two different angles in terms of my own experience. So first of all, I'm going to talk about it from the engineering side of things, right? And that's probably where the expertise is going to exist in your business or you're going to bring in some consultants to try and help you with this and all the rest of it. So you have a bunch of people who are way, way up to speed in terms of what state of the art might be from a machine learning point of view and all the rest of it. If you are in that role inside an organisation, one of the things that I've seen happen over the years, it happened with AI, it happens with other things as well, is as a technologist, if you're not talking about outcomes, your business will not listen to you. That's the very, very blunt short way of putting it is that if I turn up and go, this new thing's been invented and it can do, and you list off the features, it can identify dogs and cats and it will turn things pink and blue. And not one part of what you're talking about resonates with your customers or the people that you're trying to talk to on the business side of things. You're just going to go, what is this? This is nonsense. Like I have some problems and you have solved none of them.
So that's definitely one of the big, big kind of stumbling blocks that I've seen, innovation teams or the kind of advanced AI or machine learning teams or whatever happens to be, is that like that little bit of translate to business value can go missing. And that's frustrating for everyone involved.
You can kind of see this kind of playing out where, on the engineering side of things and the research side of things, people are getting a bit frustrated that nobody's listening to us. And then on the business side of things, it's like, look at those eejits with their toys. They're not doing anything. And that's probably one of the biggest stumbling blocks, I'd say.
Gareth Workman
Yeah, it's a self-fulfilling thing - neither party are getting what they want out of it and that just, kind of, frustration reigns across all conversations.
Rory Hanratty
Yeah. Now the other side of it, is on the business side of things, is that it's very easy to get high level pitches of why these things work. And actually one of the things that, you know, you kind of, you can kind of see happening over and over again is in technology magazines or kind of reports or, you know, Gartner hype cycle type things. These things get hyped up to a level that does not reflect their capability sometimes. So as a business, you might decide AI is the very fellow for us, we're going to take this into the future and our business is going to transform. But you have done that without actually understanding, can it do any of the things that you need to do? Are you just picking up on what people are pushing out there that it's capable of doing without actually getting into the weeds of the details? So that's the other side of that coin - is that yes, from an engineering side, you can produce all this technology that can do all sorts of stuff, but it doesn't solve a business problem.
And then what you're doing from a business side is you're going, we can use this technology to solve our problems without actually understanding, can it or even better, should it solve our problems? Cause that's one of the things as well is that, I think, there can be a little bit of FOMO, like fear of missing out here. Everyone else is doing AI, so we should too. It's not a good way to start any initiative around adoptive AI machine learning.
Gareth Workman
Yeah, it's like you have AI on a post-it note and you're just desperate to stick it onto something or you're putting it onto the right thing, as it were.
Rory Hanratty
Right. Yeah, yeah, yeah. I mean, this is one of those things is that like, you know, different companies work in different ways, but somebody could have been asked an awkward question by their board, for example: where are you using AI? And the immediate response is: let's use AI. The more considered response is: we have taken time to look at problems within our business. We have tasked our innovation, our research team, or whoever, to evaluate whether or not we can solve those problems. And we have an outcome that we're going to drive towards in terms of delivering project X or Y or Z, or whatever it happens to be, that's relevant to you as an organisation.
Gareth Workman
Yeah. Teams often can sometimes chase the hype as you say, maybe the product or over engineering solutions. Keeping AI grounded and those clear outcomes, how does that avoid that, kind of, empire building or gold plated solutions that maybe don't solve a problem?
Rory Hanratty
It's say what you're trying to achieve. And being really, really clear with your teams that the outcome we are aiming for here is X or Y or Z. So for example, in Axial3D, one of the problems we solved is this idea of segmentation of medical images. So that is taking a CT scan or an MRI, getting rid of the data that you don't need, keeping what you do, and then creating an exact replica in 3D of some human anatomy that can be used for things like surgical planning, patient education, and so on and so forth.
What we didn't do was we didn't set the challenge of 'please segment the entire human anatomy' and let's do that. And then we end up with this amazing thing that segments the entire human anatomy, but turns out actually may not be useful for any of the business challenges that we're trying to solve for some of our customers, right?
So the business problem that we solve for our customers is that manually segmenting humans for pre-surgical planning takes a lot of people and a lot of time and it's very expensive. We focus narrowly on the use cases where segmentation applies. So I don't need to segment the full human if I'm doing a knee replacement or a shoulder replacement. So we got very, very good at those anatomies rather than everything. And that's what can happen if you don't have those kind of clear outcomes or goals. Like, can we segment everything? Yes, we can. Can we segment it to a level that's needed specifically for use case A or B? Yes, we could.
But it might take an awful lot longer to get to that point than it does to narrowly focus on an outcome that you're trying to achieve. So, being really, really clear on what those outcomes are is very important. The other thing that's probably worth talking a little bit about here as well is that, as well as the kind of, outcome focus in terms of technologically, we want to achieve this and we want to automate this as well. The other bit that needs to come along with that as well is that, like we chatted just at the start is, who's going to use it? And what risks are associated with this automation that we're gonna achieve?
Because if you're not doing that, you have a real risk about introducing automation that is biased or becomes overly trusted where there might be some risks with just kind of building your entire business on the back of something that is probably non-deterministic sometimes in the way that it works. So, if you give it this image A, image B, image C, you might get slightly different results each time, if you're doing any types of, let's say, manufacturing safety checks and so on. So you need to also include that analysis as part of anything that you're doing as well. Like in software engineering and stuff, we're used to that. It's like unit testing and all the rest of it, but like that's a bit different when it comes to AI and automation. So, that's another aspect you need to think about. There's like, I'm sure people listening to this are probably getting the impression that there is a lot to this..
Gareth Workman
Yeah.
Rory Hanratty
..which is definitely the case. Organisationally, everyone needs to be prepared for the impact that this can have, which is a lot of fun. It really is.
Gareth Workman
Super. I'm going to pull on a thread, something you touched on a little bit earlier and I'd like to hear a bit more about it. So what sort of specific role do you see leadership playing in building AI-ready cultures? Is it vision, moving blockers, something else entirely? And even maybe to look further, what should those leaders be doing to proactively shape that environment and culture?
Rory Hanratty
Great question. So I'm going to start off with understanding. So as senior leaders inside an organisation, taking the time to understand what AI and machine learning is, what it can do and what it can't do, is very, very important. So actually being able to talk and understand that language is really, really important. And that doesn't mean you have to go all the way down to the bottom and understand model weights and parameters and all this sort of stuff. You don't need to do that, but you do need to at least understand what it is, what it can do, what it can't do to start off with. So educating yourself is the first kind of stage to what a senior leadership team can do. The next thing then as well is that encouraging a culture of curiosity is important here as well. I'm gonna borrow from like, I guess, product world here is: how might we? Like asking a 'how might we?' question of your teams is a really, really good way of doing things rather than just straight up going: 'I demand that we do this' or 'we must achieve whatever'.
Flip it around a little bit and ask that question of how might we, and that's gonna introduce good answers back from people within your organisation. It's gonna create a little bit more of an open approach to how you're gonna leverage things and develop things and so on as well.
And that's actually quite important here too is that if you're gonna adopt something like AI and machine learning where it's not as guaranteed in terms of the types of impact or outcome or measurable impact that you might have, you need to create a culture that allows people to say, it doesn't work or I can't do this or we can't. So that needs to be part of what you do. So flipping that around from a cultural point of view is how might we achieve this or can you help me understand how we get to A or to B or to C? That's a super important thing to include as well.
I, kind of seen, certainly here in Axial3D, like machine learning is core to what we do, but we don't stop talking about the fact that that is core to what we do. And that's very much aligned to how we're achieving our vision and our mission. And this stuff is just going back to like almost how do you run a good organisation? Like if you run an organisation that is looking to scale and looking to grow, you're going to be focused on some of these things that I'm talking about anyway, which is people side of things, a really open culture that's interested in learning and experimenting, using innovation in the right way. I could say these things and never say the word AI as part of it. That could apply to anything that you're trying to do like, should we use a new type of chainsaw? Well, let's figure that out. How might we use the new chainsaw to achieve business outcome A, B and C? You can swap in machine learning. You can swap in software engineering. You can swap in a new CRM platform.
It's the same sort of things that you would like to see from a leadership team that are looking to grow and looking to take advantage of innovation at large. The difference being is that the level of education that you need to have when it comes to AI and machine learning is maybe just that little bit different to what you would typically look at, I think.
Gareth Workman
Yeah. Yeah. And, maybe, I'm going to be at risk of getting you to repeat yourself, but where do you see where a shift in mindset has had that meaningful difference in that success of an AI initiative or other things? Maybe pull that thread a little bit for us there.
Rory Hanratty
Yeah, for sure. So, our example in Axial3D is pretty straightforward. Segmentation is really, really hard, taking way too long. This is years ago, by the way. This is going back six or seven years, I think, in Axial3D's history. How might we speed this up? Tried out some techniques using some deep learning models and discovered, oh my God, we can go quicker. And what happened there was, it wasn't, let's use AI and machine learning, was the ask. It was, how do we speed up segmentation? And that shifted the entire organisation towards this is what we do now. That's a huge, huge difference.
The thing I think there is the, kind of, idea that what triggered that was curiosity about possibility of using some different answers. I can give you another example from elsewhere that I've seen in my time on this earth. So it's very much centred around what happened, I would say, six or seven years ago when the hype was around data platforms and data lakes and we're gonna have all the data and all this other kind of stuff. I think a lot of those initiatives are probably still going. People are now starting to realise that actually there's a different way that we should be talking about those types of initiatives. But I've seen in healthcare examples, asking a question for example around how do we get better at predicting outcomes for people who are admitted into hospitals, for example. So, somebody comes into A&E, how do we better predict what outcome they're gonna have based on some certain information? The current standard today is just based on really blunt evaluations like, you know, what age is the person? What sex is the person? Have we seen them before? So the outcomes gonna be better or worse. There was a little project that was delivered in a health service where they actually opened up more data to build a slightly better predictive model than your age, your location, your sex, basically. And what happened there was by introducing this ability of tapping into new data and additional data, they found the performance of the prediction for the model went up by a significant amount, like it outperformed today's model massively. And what that did for that organisation was it didn't immediately transform how they were doing A&E admittance, but actually what it made them realise was access the data and asking the right questions will transform how we can do healthcare. And it's huge.
And what happens there is that it's like that 'Aha!' moment for people that makes a huge difference. And I can neatly link this back to the idea that outcomes are the most important thing that you can focus on when you're talking about adopting AI or machine learning. When you can say, we have just managed to increase this, reduce this, speed up that for everybody in the organisation. They look at that and go, oh, now we understand the possibility here. This is something that we can actually talk about and we can actually celebrate and we can actually look at it.
And what this reflects back to, I can feel fortunate to having been involved in this in the, sort of, mid 2010s was the, kind of, GDS transformation in UK government. They focused on users, but the other thing that was part of the kind of vocabulary that was used in the early days of government digital transformation was the idea of exemplars, where you can point at an awesome example of technology being used to make a real difference for your users. And that's the thing that makes a huge difference for any organisation when it comes to adopting any new technology, but you can use that exact same approach for AI or machine learning.
Gareth Workman
As you say, just make it very real, very understandable, tangible, not this abstract, difficult to comprehend the value one way or another.
Rory Hanratty
Yeah, yeah, like we're going to use large items as long as they increase productivity. It's like, okay, cool. Starting to fall asleep now. Show me how. Give me a real example that I can point at. And then when I'm talking to somebody in business unit two, or I'm talking to somebody else in the other part of the organisation, instead of going, we're going to improve productivity by using large language models. And nobody knows what that means. You can say, we have reduced the time that it takes to write reports on customer satisfaction by this amount, or we're able to discover this new information about how our users use our system or whatever it happens to be. But when it's real and tangible and it's an outcome that matters, that's when you'll see people starting to go, right, this is real. This means something to me. This means something to us. We could do amazing things with this.
Gareth Workman
Very cool, very cool. So maybe looking a bit further ahead. So again, what advice would you give to leaders aiming to embed that sort of sustainable future focused mindset across their business? You know, if they're starting here today, listening to you and going, where do we start, Rory?
Rory Hanratty
Wow, that's a tricky one. I think start with understanding problems in your business today and start looking at things with a 'how might we?' kind of an approach. And as well as your typical tools that you're going to bring into the organisation to figure out 'how might we?', you need to have that knowledge and understanding of some of the capabilities that AI or machine learning turn up with, and bring that into the set of tools that you might apply to try and solve your problem. That's the kind of simplest starting point.
Now, obviously there's an awful lot behind that. If we don't understand what the tools can do, how do we become educated about it? Great, that's brilliant. You're starting to build a plan and you're starting to build your strategy around, hey, AI can help your business.
I remember, this is an interesting one, I found a presentation that was from 2016, that I did for a number of business leaders in Northern Ireland, and it was around AI and how to develop a strategy. And my single statement around what should we do about AI in terms of strategy, and it was simply: have one. And I think actually that advice probably still holds for quite a few people today. Back when I made that statement was before large language models took off and the hype cycle just went crazy. This was when you could actually achieve real things with plain old machine learning. And now with this acceleration of what Google are making available, Microsoft are making available, Amazon are making available, when you've got OpenAI, you have Claude and Anthropic, these are all tools that can be adopted into businesses to increase productivity now. And if you're not thinking about how might I be able to use them, you can guarantee that other people out there, who are doing the same thing, are gonna have a bit of an advantage over you. So that's the other bit of advice, if you don't have an AI strategy, have one.
Gareth Workman
And it might seem really simple, but as you say, that North Star and a bit of guidance helps galvanise people for them to get behind it as well. It gives them something to focus on and a direction of travel, as it were.
Rory Hanratty
Yep, 100%, 100%. Anchoring it in your context and what you're trying to achieve as a business and what you're trying to be. And it could be that AI has minimal impact, but enough to make it worthwhile to bring into your organisation. It could be that it's utterly transformational and opens up completely new lines of business. It's really fascinating.
Gareth Workman
So at Beyond Boundaries, we just don't dive into the future of AI, we aim to give business leaders, practical insights they need to act today. So, Rory, if a senior leader asked you if you could live a moment again in terms of a lesson learned, what would you tell them?
Rory Hanratty
So actually, I'm going to go back in time. One of my first spins around the block around trying to encourage adoption from AI. I think one of the mistakes that we made back then when we were trying to do this was we were focused a little bit too much, honestly, I'm a technologist by background, right? So I'm going to be biased a little bit towards this, but I was very much focused on the technology, trying to run towards and convince up from a business point of view, this is why this technology is amazing and it's awesome and all the rest of it. And I did not do a good enough job of framing it in terms that my business partners would have understood or listened to. So, I think for me as a technologist, like if I was to go back in time and go, here, you need to do a little bit more of this kind of framing in terms of problems, 100% would have done that.
I think on the other side of the fence as well, actually it's a real personal story for me, is that from a business point of view, having a handle on the types of problems and challenges that you are facing and having regular conversations with your innovation teams or R&D teams and making sure that you're actually both aligned. So, this is more for the like CEs and CIs and CFOs who might be listening to this conversation, is really thinking about that. Like, are you having focused, outcome-oriented conversations with people who are providing innovation for you in your business? And are they helping to work with you and you are a team? That's thing here as well. This isn't an over the fence type thing. It's like we have a problem, you go solve it and they go, we solve it, we chuck it back again. As a team, are you joined up on what you're trying to achieve? So that would be it. That's my big one. That's the one I've learned from my own failings in that department, I would say.
Gareth Workman
Fabulous. So look, we've covered a lot of ground today, you know, covering critical factors like AI adoption, cultural transformation, leadership strategy. So as we wrap up, Rory, if there's a key message that you wanted business leaders to take away today, what would that be?
Rory Hanratty
Outcomes. What are you trying to do? What are you trying to get to? Right? What are you, what are you in the business of? And how are you trying to get to those outcomes? That's it, like start from there. Start from, we would like to achieve this or this, or whatever it happens to be. Start from there and go, how might we solve that problem using chainsaws, tractors, software, AI?
Gareth Workman
Yeah, fantastic advice. So, that brings us to the end of this episode of Beyond Boundaries. Rory, thank you for a really inspiring conversation. It's clear that building AI-ready culture starts with the right mindset and a focus on people, not just technology.
Rory Hanratty
100%. That's the most valuable thing with anything is the conversations that you have around how you achieve your goals. All of the other stuff will fall into place once you're doing that right.
Gareth Workman
Thanking you.
For our listeners, the takeaway is simple. If you want AI to make a lasting impact, start by investing in your people and the culture that supports them.
At Kainos, we're committed to helping you understand and leverage the transformative power of AI while navigating the challenges of ethical and responsible tech adoption. So if you enjoyed today's conversation, make sure you subscribe to wherever you get your podcasts and head to kainos.com/beyondboundaries for more insights.
Join us next time as we continue to unpack the big questions shaping AI and business. So until then, stay curious, stay innovative, and let's go Beyond Boundaries together!
End of episode