Chapter six: Conclusions
A parallel between sustainability and technology ethics
In the past two decades, sustainability has moved from being a less recognised aspect of business to perhaps the most dominant commercial narrative in history.
Where, before, chief sustainability officers were rare, now there’s barely a large corporation on the planet that doesn’t have one.
The more investors and consumers have become critical of the effects of a carbon-intensive “business as usual” approach, the more companies have responded with environmental sustainability initiatives, hires and commitments.
Although the impacts of artificial intelligence are arguably lower down on the list of critical societal challenges, some lessons from corporate sustainability movements can help to prevent the challenge of trust in artificial intelligence from escalating to the state of crisis we have seen with climate change.
“ESG will grow to include the artificial intelligence vertical and data governance, because they are core governance issues.” Liz Grennan believes; having seen that algorithmic impact assessments, privacy programs and data officers are becoming necessary around the world.
Specifically, trust in artificial intelligence can be supported by the same sort of actions that businesses are using to demonstrate their environmental sustainability within the ESG frame: the mechanisms, commitments and strategies discussed in this report.
For David Leslie, the challenge is clearly present for both environmental sustainability and trustworthy artificial intelligence. What is needed, he contends, is a shift in focus towards real democratic governance:
“Why are companies still polluting the environment? Because there's a higher level of accountability to the boards and to optimising profitability than there is to real democratic governance of the corporate practices, and the same will be true in the artificial intelligence ecosystem. This is to say that the more that you have inclusive involvement of impacted stakeholders in the decision-making mechanism, true democratic governance, the more transformation you will see in the practices themselves.”
Leslie thinks that we are at a formative stage in the way we design and govern artificial intelligence systems. We have yet to see an ‘ex ante’ mindset emerge – that is, not thinking about how we can make existing artificial intelligence systems more trustworthy, but how we embed ethical design practices into teams and products from the outset to achieve greater trust in those systems. This mindset, he says, is ultimately going to come from a “shift of culture towards the democratic governance of technology”.
The three hypotheses: a call to action
1. Responsibility is not only a role
The ethicist, despite being a pivotal steward of ethical artificial intelligence, shouldn’t be seen as the only solution to establishing trust.
Much like the effect that public concern about climate change has had on environmental sustainability practices, if the artificial intelligence ecosystem is to reach a point where an awareness of ethical harms can exist within all development practices, an organisational culture in which everyone has an ethicist’s perspective seems crucial. The responsibility can’t remain siloed within the remit of an individual.
Ultimately the role of the ethicist is likely to become more and more taken for granted as the procedures become normalised. Nonetheless, if we are to realise the full potential of artificial intelligence to transform the world for the better, the responsibility (not just the role) of ethics needs to become more and more embedded.
Companies can begin by seeking out ethics professionals and giving them the remit to change the way the responsibility for ethical development is communicated and addressed. Executive and board level sponsorship of ethics initiatives is also crucial. This makes the subject of ethical application much more visible, and puts it on the same footing as other key organisational objectives. Ask who is accountable for driving this initiative forward, and how they will be quantifying and reporting on progress; many of the mechanisms discussed in Chapter 3 of this report are not only ready for adoption right now, but also provide measurable outputs.
2. Standardisation from diversity
While there have been tentative steps towards international standardisation and certification, there is insufficient coordination between the many movements to bring consensus on ethical best practices for artificial intelligence.
However, given the imminence of enforceable legislation, being familiar with such international standards and recommendations, which often emerge from a diversity of perspectives and cultures, will provide organisations with adaptability to forthcoming regulatory requirements.
Companies should be familiar not only with the work in this space, but also with the specific standards that apply to their sectors. As industry specific standards and compliance frameworks become commonplace, a level of organisation competence to translate them into everyday processes will be needed.
3. Explainability to understandability, prospect to procedure
Attempts to provide a technical explanation of complex algorithmic behaviour has yet to produce well-placed trust in artificial intelligence systems.
The mechanisms of understandability that emphasise context and pave the way for transparency between stakeholders have similar characteristics to the reporting now used in environmental sustainability, although the disclosure of ethical harms from artificial intelligence remains further behind.
In both spaces, investor and consumer attention is focusing on potential harms. Short to medium-term business value can still be realised but, in the long term, reporting on ethical or sustainability-related impacts must be just as important as financial disclosures. A range of mechanisms can enable well-placed trust, and an understanding of what users and customers really need is crucial to deciding which are most appropriate; whether it’s social context, technical explanations, transparency statements, certification or all of the above.
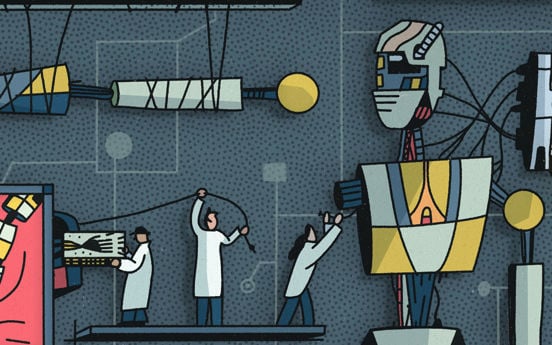
What this might mean for trust?
Both the cultivation of cross-organisational ethical responsibility and the inclusive approaches to standardisation, touched on in sections one and two, can help foster trusting relations among teams that develop AI. They also offer a greater overall trust between the public and artificial intelligence companies.
The practices and mechanisms described in the third section pave the way for a similar kind of trust. Yet trust from multiple stakeholders is difficult to maintain and there is a danger that these practices will provide only a layer of trustworthiness.
Perhaps the crucial transformation for the artificial intelligence ecosystem will come from a paradigm shift in which democratic governance of each individual system is embedded by design. Only then will AI systems realise their long-term business value and gain the well-placed trust of all those who shape and are shaped by them.