Chapter two: Starting out and understanding the risks
Starting out: Trust and maturity
We investigated 10 different examples of models and indices used to evaluate the maturity of a company’s artificial intelligence efforts. From looking at leading companies such as IBM, Dataiku and AppliedAI, it’s clear that even at different stages of maturity, companies do have some things in common.
The frameworks distinguish generally between lower, middle and higher levels of maturity.
The categories aren’t completely separate, and some models use different criteria, reflecting the complex process of adoption. But we can draw from them some key ideas and attributes to help understand how maturity changes, and what that might mean for levels of trust:
- Lower level maturity – often termed “experimenters”, “dabblers” or “initialisers” – is characterised by businesses that are starting out on their first AI projects, have not yet created a clear business case for further investment and are only beginning to realise value through artificial intelligence.
- Middle level maturity – often termed “explorers”, “practitioners” or “expanders” – is characterised by businesses that have deployed artificial intelligence and are driving value in some way, but have not yet done so at scale.
- Higher level maturity – often termed “leaders”, “shapers” or “experts” – is characterised by businesses that have created significant value through the use of artificial intelligence at scale, and continue to innovate.
Maturity varies not just between companies but also within them. Efforts to use artificial intelligence in one area of a business might be more advanced or effective than in others. But, whether businesses are large or small, mature or immature, digitised or digitising, they can all investigate trustworthiness.
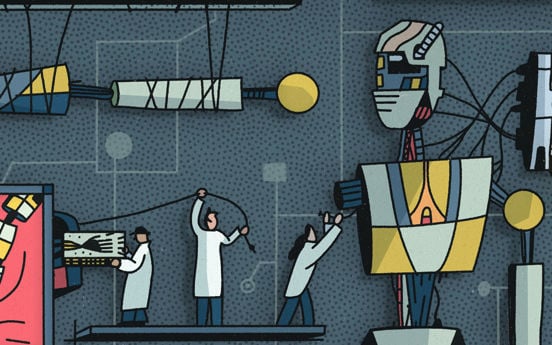
Understanding the risks: harms from misuse of artificial intelligence
In an increasingly data-driven world, complex algorithms are being used as business solutions in a huge number of commercial domains.
“Particularly when systems are poorly designed and tested, the deployment of some solutions can lead to and perpetuate systemic harms,” Peter Campbell, Data & AI Practice Director at Kainos, told us.
These harms can often emerge from building on and reinforcing racial, gender or other socio-economic biases – though this shouldn’t be framed as a problem inherent in artificial intelligence itself. They are down to poor design, governance and implementation. Here are some recent examples:
- Three commercial applications of AI-driven facial recognition technology were found to perpetuate racial and gender-based discrimination, as shown by Joy Buloamwini, of MIT Media Lab, who revealed the systems had low accuracy in detecting women of colour.
- Some Uber drivers were denied access to, and in some cases removed from, the company platform, harming their livelihoods, after algorithmic decision-making systems wrongly removed them because the authentication software failed to detect their faces.
- In 2017 it was revealed that Google’s search algorithm was generating search results along discriminatory lines, with the algorithmic autofill suggestion for “does Islam…” adding “permit terrorism” to users’ searchbars. While recommendations of a Google algorithm are a reflection of historical, social, cultural and technical factors, and although it was clearly not Google’s intention to make discriminatory recommendations, producing such harmful content was still a function of the algorithmic system.
- Socio-economically discriminating algorithms were found to be used by Italian car insurance companies. A study of the opaque algorithms used to create insurance quotes found that rates varied according to citizenship and birthplace, with a driver born in Ghana being charged as much as €1,000 more than a person with an identical profile born in Milan.
Organisations can’t bury their heads in the face of these challenges - “we are no longer in a Wild West”, as Adam Kovalevsky, VP of Product at Workday, told us.
It’s not just in sensitive areas such as insurance, financial services and healthcare that these harms present a serious issue. Given their widespread presence, two critical questions arise:
- How can we make sense of algorithmic systems, both in design and use, to hold people accountable for their function?
- How can we make sure that algorithmic systems are ethically designed, to mitigate harms before they arise?
By addressing these two questions, of accountability and responsibility, this report shows why it's important to explore how trust in artificial intelligence is created, and how it can be sustained in the future.
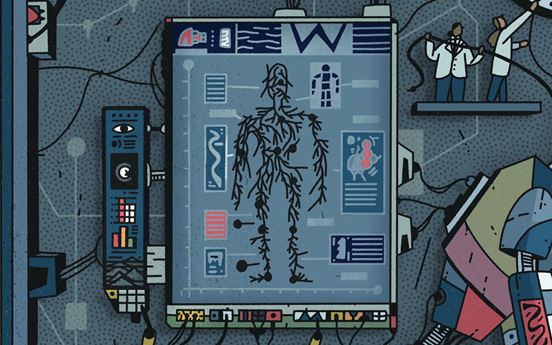
Three hypotheses about the future of trust in artificial intelligence
Drawing on interviews conducted with experts at Accenture, the Alan Turing Institute, UNESCO, Sky, IEEE and other leading organisations, as well as a review of the most recent literature on ethical and responsible technology, we present three hypotheses, each shedding light on the ways that trust can be established and refined within artificial intelligence ecosystems.