Chapter five: Hypothesis three - From explainability to understandability and prospect to procedure
Technical explainability hasn’t enabled trust, but a number of overlapping procedures are emerging as helpful alternatives
Opening the black box: a lost cause?
Commercial artificial intelligence relies on complex processes. Whether it’s advanced statistical modelling, machine or deep learning techniques, decisions are being made using very complicated maths.
In a blog post, Faculty, a leading AI solutions provider, suggests that there is often a correlation between complexity – the inner working of the so-called algorithmic “black box” – and the performance of the system: the more complex and seemingly unexplainable the behaviour of the model, the more accurate it can often be.
There’s widespread acknowledgement that poorly designed decision-making systems can lead to unsafe and potentially harmful machine behaviour, often stemming from human biases that may reflect racial, gendered or socio-economic differences. Observatories, like the one maintained by the Eticas Foundation, can help us to trace these harms.
Given their mathematical complexity and societal importance, there have been many efforts to explain algorithmic decision-making systems. Academics, industry practitioners, government organisations and NGOs have tried to explain the behaviour of such systems. This is known as explainability. The logic of explainability is that the behaviour of algorithmic decision-making systems should be justified and subject to scrutiny so that the societal impacts can be more successfully managed by both businesses and governments.
Calls for explainability have been spearheaded by global technology companies and academic researchers through the development of technical methodologies and toolkits that are often grouped into a sub-discipline known as explainable AI (XAI).
XAI emerged around 2017 to help AI practitioners explain complex model behaviour to other practitioners: IBM has an AI Explainability 360 Kit which provides eight “state of the art explainability algorithms add transparency throughout AI systems”; Google has developed Explainable AI to provide “human-interpretable explanations of machine learning models through its tools like “AutoML” and “Vertex AI”; and Microsoft has its Interpret ML tool to analyse models and explain behaviours.
Yet, while these explainability techniques profess to “grow end-user trust and improve transparency”, as Google claims, the link between giving a technical explanation and creating trust is not always clear.
Jenn Wortman Vaughan, a Senior Principal Researcher at Microsoft Research, told us:
"Providing explanations can cause data scientists – as relative experts – to overtrust machine-learning models. What we should be aiming for is appropriate trust: how can we boost stakeholders’ trust in the system when it is doing the right thing, while fostering an understanding of the limitations of the system and what can potentially go wrong?”
Similarly, trust expert and Research Professor at the University of Coventry, Dr Frens Kroeger, told us that for most stakeholders in the artificial intelligence ecosystem, the technical tools of explainability don’t necessarily provide appropriate or well-placed trust.
Talking about the way that explainability has developed, Kroeger suggested that much of it “was just purely hard technical explanations… where software engineers interpret them and then you say, ‘yeah, okay — that’s trustworthy’”.
“But the explanation doesn't make sense to a vast majority of the people out there. Because they're not experts.”
The sense we got from Kroeger was that there is a need to go beyond just technical explainability tools if we want to encourage well-placed trust in AI systems: “Can we get away from technical explanations and can we try and devise social explanations?”
Instead, he prefers explanations that reflect “the sort of institutional framework that surrounds the development of artificial intelligence; that is:
“How can we develop an explanation that can in some way make the values of those companies that are behind it a bit more tangible?”
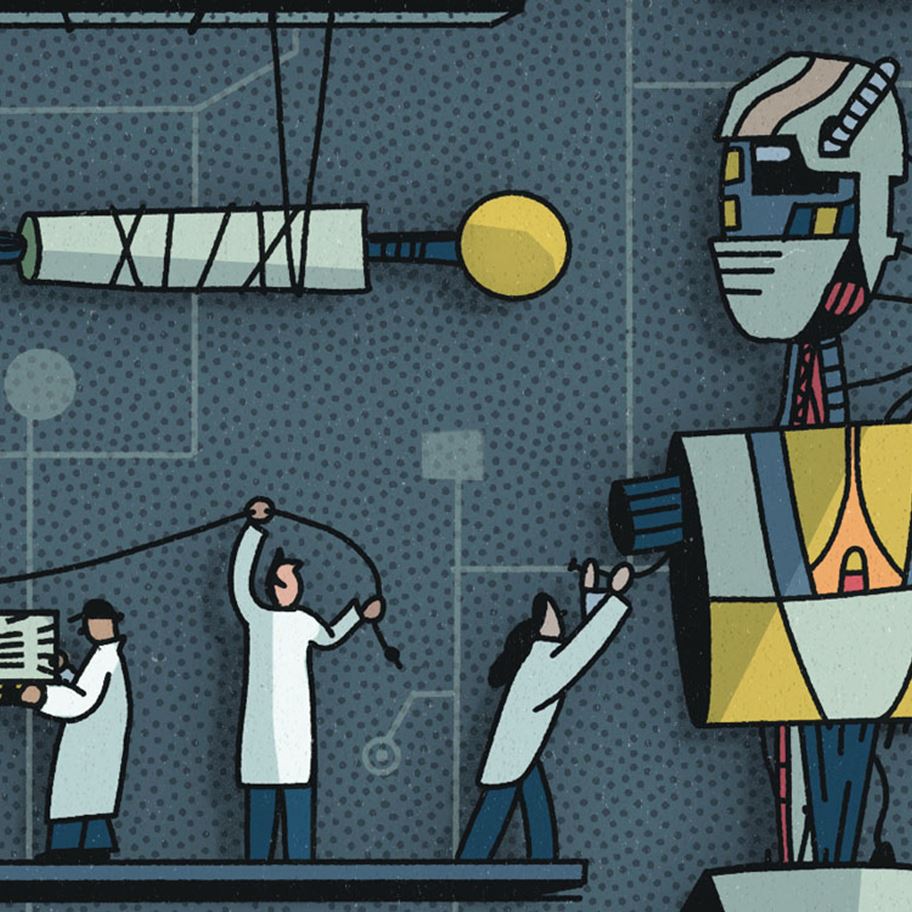
Kroeger calls it expanded or social explainability, a phrase that puts the focus on explaining the social contexts and values that surround algorithm development and deployment decisions. It’s an idea that echoes two of the four pillars the ICO and the Alan Turing Institute gave for their guidance on explainability, notably, “consider context and reflect on impacts”.
Towards holistic explainability
Following a similar line of thought, David Leslie told us about his work as lead researcher on the joint ICO and Alan Turing initiative Project Explain. He talked us through what he calls a “topology of explanations” to “do explainability from a more holistic point of view”.
Beyond what Leslie calls the “rationale explanation” (the technical component that explains the function of an algorithm), the multitude of approaches within this holistic explainability include:
- Impact explanations: “Have you built in mechanisms for making sure that impacted stakeholders will be privy to explanations of how the ethics has been done and what decisions have been made and the deliberation behind those kinds of choices?”
- Data explanations: “Being clear about the provenance of the data set.”
- Fairness explanations: “Being able to demonstrate across the design, development, deployment lifecycle that a project team has sufficiently considered potential pitfalls of bias, and that there has been a deliberate and transparent approach to defining what fairness criteria are being incorporated into the system.”
- Responsibility explanations: “Being transparent about who, at any given point across the lifecycle, owns and is involved in the decision-making”
So beyond the technical explainability, “there's all these other kinds of needed explanations that can justify public trust in the system”.
This speaks to the need for context-specific transparency highlighted by Jenn Wortman Vaughan:
“There are different stakeholders of AI systems who require transparency, including data scientists building systems, regulators, decision-makers using AI systems, and those who are ultimately impacted by the systems. These stakeholders have different goals, different expertise, and therefore different needs, so the approach that works best for one may not be helpful for another.”
She details some of the different stakeholders and the transparency they need:
- For technical practitioners: “If we’re thinking about a data scientist trying to debug a machine-learning model, they might benefit most from a tool like InterpretML which provides [both specific and general] explanations of the model’s behaviour.”
- For business stakeholders: “A decision-maker who is trying to determine whether or not an AI system is appropriate for their company may be better off with a clear description of the intended use cases, capabilities and, perhaps most crucially, limitations of that system. This is what we designed Microsoft’s Transparency Notes for.”
- For regulators or compliance officers: “What’s needed may be an understanding of the characteristics of the particular dataset that was used to train a model, in which case a datasheet may be most appropriate.”
A thread that runs through the work of Leslie, Kroeger and Wortman-Vaughan is a focus on the people and contexts surrounding AI development – what some experts have called “meaningful transparency”. Meaningful transparency, as a blog post from the Ada Lovelace Institute notes, is what gets us to a place of “genuine understanding and engagement with algorithmic processes” by pulling the social and policy dimensions of AI development decisions into focus.
Meaningful or contextual transparency, holistic or social explainability – some may think it’s all just semantics. But there is a common theme here: engaging the people and contexts beyond the technical components of a system can promote understanding of, and well-placed trust in, artificial intelligence.
Making it more understandable: the procedural toolbox
Many of the experts we spoke to have been involved in creating procedures to promote well-placed trust in AI architects and products. They have done so in ways that echo Leslie’s holistic characterisation of explainability. Some of these procedures are still evolving, and there was a consensus that there isn’t one silver bullet to solve the problem of trust, but many complementary approaches.
Nonetheless, being familiar with these developments now is an advantage to any organisation developing trustworthy AI practices, depending on where they are on the maturity spectrum.
Decision Documenting
Lisa Talia Moretti, for example, suggested that a process of decision documenting was crucial to enable the social transparency of AI workflows. This might be:
“A single user researcher or a single anthropologist working within the team whose sole job it is to actually document the way that the team actually went about this and document that decision-making process. Or you could do something more collectively where you have a team who maintain weekly notes and have a constant trail of meetings around an AI product decision; noting in a few lines that this is who we spoke to, these are the decisions we made.”
Sharing how decisions are made can be a key driver of trust internally and externally, according to a project led by the Partnership on AI called ABOUT ML. A blog post from ABOUT ML suggests that “the process of documentation can support the goal of transparency by prompting critical thinking about the ethical implications of each step in the artificial intelligence lifecycle and ensuring that important steps are not skipped”.
But how documentation is used and what explanations it provides can vary according to who it is used for, as Beena Ammanath told us: “Each stakeholder requires different levels of explanation for the AI solution.”
One sector in which explaining algorithmic decisions is a crucial responsibility is in defence applications.
We spoke to Air Cdre David Rowland, the Senior Responsible Officer for the Ministry of Defence’s new AI Centre, who told us that although the MoD currently doesn’t use artificial intelligence in high-stakes contexts, the need for explaining decisions is massive “because of the nature of what defence actually does”:
“A lot of what defence does is deterrence and sub-threshold cyber type activity to show those that could potentially do us harm how strong we are in that environment – AI will play a part in that future.”
“Some of it is just to show them that they can’t attack our network so they can’t commit fraud against us, or for our workforce not to jump on the wrong links…
“But of course if that all goes wrong then we do need to create violence and harm against those that would do us harm…
“Therefore, it's absolutely incumbent upon us that we make sure that if we have got life and death decisions, then we absolutely understand the mechanisms involved in those decisions.”
Processes of decision documenting clearly have a crucial place in many levels of ethical and safety-related risk.
Explainability statements
Where the process of decision documentation is used to report internally on how technical and strategic choices are made in a system, an explainability statement can help inform external users about the AI used in algorithmically supported platforms.
Minesh Tanna, Managing Associate at Simmons & Simmons, introduced us to an explainability statement – the first of its kind in the world – that he worked on for a health management and self-care app called Healthily. The statement provides users with information on “how the artificial intelligence in our app works, including when, how and why we use this technology”. HireVue, the leading HR interview platform, has since followed on with a similar document, also reviewed by the UK’s Information Commissioner’s Office under the aegis of their implementation of the GDPR’s rules on automated decisions..
Tim Gordon, co-founder of Best Practice AI, an AI Management Consultancy who partnered with Simmons & Simmons on the Healthily work, suggested in a recent blog post that explainability statements might require some work – providing transparency on data sourcing and tagging, and showing how algorithms are trained and what processes are in place to respond to and manage harms is not necessarily a simple task.
But he sees five reasons why businesses should consider preparing one:
1. The legal expectation: In Europe, under GDPR, you need to be able to explain how, where and why you are using AI.
2. Internal organisation: It brings stakeholders together to make sure nothing “slips between the cracks”.
3. Customer value: It provides detailed information for those who want to know about how algorithms are used. An AI Explainability Statement provides the material to generate even one-page summaries - as for example HireVue has done from their work with Best Practice AI.
4. Limits liability: Court cases in Europe, such as those against Uber/Ola in the Netherlands, have set clear precedents that you need to explain what is going on if AI is affecting individual workers.
5. Growing international expectation: With regulation in China, New York and California moving in the direction of transparency, explainability statements are globally relevant.
He argues that ultimately the investment in transparency is the path to generating trust.
One-pagers, leaflets and Nutri-Score labels
A 13-page explainability statement may not seem that long compared with the several hundred pages of AI ethics principles that some organisations have published. Yet to an end-user with a relatively low – even non-existent – knowledge of artificial intelligence, it may be optimistic to think such a document can offer much value.
At the other end of the spectrum, the one-page toolkit developed by Rolls-Royce, known as the Aletheia Framework, provides a practical guide for developers, executives and boards before and during usage of artificial intelligence. Rolls-Royce’s Head of Service Integrity, Lee Glazier, who spearheaded the development of the Aletheia Framework, said it was a response to a wave of long and impractical ethical frameworks that emerged just over two years ago.
Instead they created an “A3 sheet of paper, that is really agile and developers can fill it out really quickly”, he explained.
Going beyond understanding an AI system, the framework asks those developing and deploying AI to consider “32 facets of social impact, governance and trust and transparency and to provide evidence which can then be used to engage with approvers, stakeholders or auditors”.
In terms of explaining the algorithmic behaviour of a system, the framework adopts what Caroline Gorski, the Group Director at Rolls-Royce’s R² Data Labs, calls an “input-output assurance model”: “It ensures the inputs are trustworthy and outputs are trustworthy; it does not require you to publish every element of the model in between.”
Gorski’s reasoning for this approach echoes the concern that was relayed over and over again during the interviews we conducted:
“It is profoundly difficult to explain those black box models. While there is lots of good work on this, in our view it is probably several years, if not a decade, away from being possible.”
This type of document has practical value, and the framework is now available open-source following interest from organisations in many other sectors.
It has made an impression, Glazier says. “It was deemed, even by big tech and our peers, as something that was unique because it was accessible and it was an A3 sheet of paper, rather than a 100-page document.”
We saw a similar practicality in the “algorithmic leaflet”. The team at Eticas Consulting, led by Gemma Galdón-Clavell, is developing the leaflet with several governments for use alongside their labour algorithms.
“Taking the idea of the medical leaflet for when you buy medicine: it comes with a document that tells you how to consume that medicine in conditions of safety. It tells you about the ingredients that go into it and you don't always understand everything. But it's a document that helps regulators and the public understand what some of the impacts of that piece of medicine are.”
For an even more user-friendly form of explanation, her team is working on Nutri-Score labels to provide a “visually comprehensive way of understanding” and “comparing between different products offered in algorithmic decision-making… like in Europe where you have an ABCD system for kitchen appliances”.
Algorithmic impact assessments, audits and assurance
Being “historiographers” about ethics and trusting artificial intelligence, says David Leslie, it’s clear “there have been various waves, from principles to practice to building assurance mechanisms”.
The mechanisms discussed so far in this section provide a few options to build multi-stakeholder trust that go beyond the first stages of this ethical AI journey, by focusing on the understandability and social transparency of AI development and deployment.
Yet to help reach a critical mass of public trust across the AI ecosystem, many of the experts we spoke to are working on initiatives that provide assessment, reporting and assurance of ethical and responsible AI practices and impacts.
Understanding a system and the decisions that shape that system is one side of what you could call the “trust-through-transparency” coin. On the other side of that coin there is the need to hold those involved with the development and deployment of that system accountable for those processes and decisions.
One “emerging mechanism” to build algorithmic accountability, says the Ada Lovelace Institute in a recent report, is the Algorithmic Impact Assessment (AIA). In a recent report from Data and Society, the authors suggest that impact assessments offer a “means to describe, measure and assign responsibility for impacts without the need to encode explicit scientific understandings in law.” They go on to suggest that the widespread interest in AIAs “comes from how they integrate measurement and responsibility”. Drawing on both sides of our trust-through-transparency coin, “an impact assessment bundles together an account of what this system does and who should remedy its problems”.
But as the Ada Lovelace also notes in its report, “AIAs are not a complete solution for accountability on their own: they are best complemented by other algorithmic accountability initiatives, such as audits or transparency registers.”
Audits are increasingly talked about among ethical AI experts. Although by no means at a stage of mainstream adoption, they are being proposed as the next step in the trust trajectory. Why? The thinking is that they could provide assessment and reporting processes to make algorithmic systems more accountable, creating a rigorous ecosystem for assuring that systems are developed in ways that are deserving of trust.
Although audits are frequently proposed using a comprehensive methodology such as that developed by Gemma Galdón-Clavell at Eticas Consulting, there are some existing tools, such as IBM’s AI Factsheets and Google’s Model Cards for Model Reporting, that companies could integrate into an AI workflow to provide the documentation and reporting that would support an audit.
Ultimately, the overarching goal of an auditing process, says Emre Kazim of Holistic AI, is to “improve confidence in the algorithm, ensure trustworthiness of the underlying system and convey both with a certification process”.
And although there don’t appear to be any concrete examples of what that certification will look like, the idea that audits can enable trust was underlined by Galdón-Clavell, who has been doing audits now for three years:
“Algorithmic audits are one of the most practical things we can do in terms of increasing trust in AI…it’s about taking back control.”
The audit can provide a practical component to the kind of AI assurance ecosystem that is mapped out in a recent report from the UK government’s Centre for Data Ethics and Innovation (CDEI). The CDEI suggests that AI assurance services, such as certain forms of audit, will help to verify independently the trustworthiness of AI systems; trust here hinges in part on the quality of the system and whether companies “do what they claim in the way they claim''.
The CDEI sees assurances playing a “critical role in building and maintaining trust as artificial intelligence becomes increasingly adopted across the economy”. Indeed, the report optimistically suggests that an industry may grow around assurance comparable in size to the market for cybersecurity, which generated some £4 billion for the UK economy in 2019 and employs 43,000 people.
In terms of regulation, experts have pointed to the audit as a critical measure to achieve compliance with legislation that appears to be on the horizon following the EU AI Act. Yet, as Adam Leon Smith’s comments from the previous section suggest, the cost of compliance could be massive, with audits involving expensive preparatory work before an auditor even comes in.
Yet it’s a necessary cost, he adds. Just as with other industries such as financial services, this is what doing business often entails.
“[If] I'm making a change to critical banking infrastructure, I have to be ready for an audit as well. You know, I have to have huge amounts of evidence of what I've done and why I've done it. And that's the cost of doing business in that space. I think because of the risk introduced by AI, this is just part of the cost of doing business.”
And yet…
There is some debate about whether the audit actually does enough in terms of addressing the risks and harms that stem from poorly designed artificial intelligence. As David Leslie warned: “I don’t think we are there… and there is a risk that audits lead to a surface-level demonstration that doesn’t address underlying problems.”
For Leslie, such surface-level mechanisms are born from what some call an ‘ex-post’ approach to technological innovation – that is, where mechanisms that address the trustworthiness of a system are drawn on after the development itself has taken place.
The danger seems to be that no matter how advanced certain mechanisms for disclosure and assurance are, they can often be taken as an end in themselves, rather than a way of reflecting an ongoing compliance and deep-rooted alignment with ethical principles.
A sceptic might point out that the increasingly mature set of environmental sustainability disclosure frameworks, such as those from CDP, don’t address the underlying issues of carbon intensive business and emissions. According to this view, despite the looming disaster of climate change, businesses haven’t moved far or fast enough to address them.
Gemma Galdón-Clavell, whose work has largely been focused on conducting algorithmic audits, acknowledges the criticism of audits that don’t go far enough. Yet she also told us that the audit methodology of Eticas emphasised an “end-to-end consideration”, looking “not just at the technical properties of a system, but the issues of power redistribution and social inefficiencies that are found alongside it”.
The audit process the Eticas team carries out often has the potential to change how teams understand their algorithms and the risks associated with them, rather than simply reflecting the characteristics of the algorithms themselves.
“When we get in, everything is disorganised: often no one knows where all the data comes from. Whether it's legal to use it, what it's doing, no one's defined the protected groups. So we need to do a lot of work together and that becomes a learning process for them.”
The audit, as a consultation process, is more than just a tick-box compliance exercise. Galdón-Clavell feels it can instil a sense of ethical awareness and responsibility in a team. “We are changing that team and we are changing the view of organisational responsibility and awareness around those issues and the role that they play.”
Eticas is one of the only organisations actually doing algorithmic audits right now. Peter Campbell of Kainos points out that such a rigorous, externally mediated approach to identifying, reporting and assessing ethical harms may end up being a challenge to scale.
Nonetheless, the audit provides perhaps the most comprehensive example of “trust through transparency”, and there is reason to think it will be a key enabler of well-placed trust among stakeholders within and beyond an organisation developing an algorithmic system.
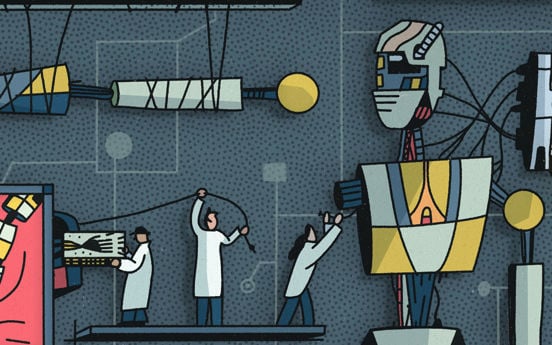
Looking ahead…
The documenting and disclosing of harms and risks – often known as negative externalities – is an established financial reporting practice across almost every sector. What is more, frameworks for both internal and external auditing and assurance that hold organisations accountable are nothing new.
Environmental harms, particularly those relating to carbon emissions, are becoming a more central part of financial reporting. Indeed, the International Sustainability Standards Board (ISSB) has recently become one of two pillars for the International Financial Reporting Standards (IFRS) to provide “investors with transparent and reliable information about a company’s financial position and performance, as well as information about sustainability factors that could create or erode its enterprise value in the short, medium and long term”.
For example, KPMG suggests that there is increasing pressure from investors and consumers to coordinate financial and non-financial statements about climate-related impacts. That is, there is a growing need to show the link between statements that are found at the top of annual reports – where strategic corporate decisions such as “net-zero targets” might be documented – and the assumptions that inform the financial statements further down.
There are even moves to integrate negative environmental externalities into financial modelling in the form of impact weighted accounts.
This movement towards a forward-looking integration appears to complement the kind of corporate sustainability envisaged by David Leslie; anticipating potential harms and risks by building contingencies into models from the start is a good way to implement sustainable practices from the outset.
On artificial intelligence we are yet to see such progress on reporting ethical risks and harms, let alone its integration into corporate financial accounts and strategy. Yet corporations can take note: it is possible that ethical and trustworthy AI will follow a similar path of integration into financial reporting to that being taken by environmental sustainability.
Even before the artificial intelligence ecosystem reaches that point, the evolution of reporting and documenting procedures is promising, from actionable processes such as decision documenting and explainability statements to more comprehensive and wide-reaching methods such as algorithmic audits and assurance framework. These processes can foster a similar culture of transparency and accountability to that seen in environmental sustainability. This bodes well for the trustworthiness of artificial intelligence at scale.
Crucially, as the use of high-risk and complex algorithms continues to increase, and transparency-enforcing legislation materialises, the need for the end-to-end auditing support that Gemma Galdón-Clavell and her team provides will become more and more important. As she tells us:
“I think that in the next five years, if we take into account all the trends and add in the avalanche of legislation at the EU level, I think that there's going to be a lot of change…. Does that mean that in five years we'll be able to just audit as a financial auditor would? Just go in and verify that things work, and then get out? That may take a bit longer. But I think that significant change is pretty much around the corner.”
What kind of change might that be? Well for the UK government’s CDEI, that change is manifested in its vision of auditing and assuring artificial intelligence – and the message is clear: “The UK will have a thriving and effective AI assurance ecosystem within the next five years.”
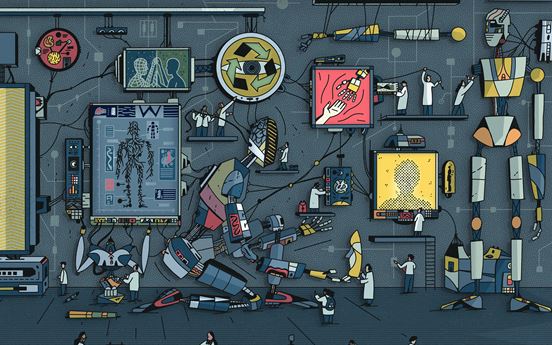
The key points:
- Engaging beyond the merely technical components of a system enables understanding of artificial intelligence, and can encourage well-placed trust in it.
- The deployment of AI involves a range of ethical risks. Particularly in settings where those risks are high it is critical to understand not only how the system behaves but also how decisions that shape the system are made, and what mechanisms are involved in those decisions.
- It is possible that ethical artificial intelligence will follow a similar path of integration into financial reporting to that being taken by environmental sustainability.